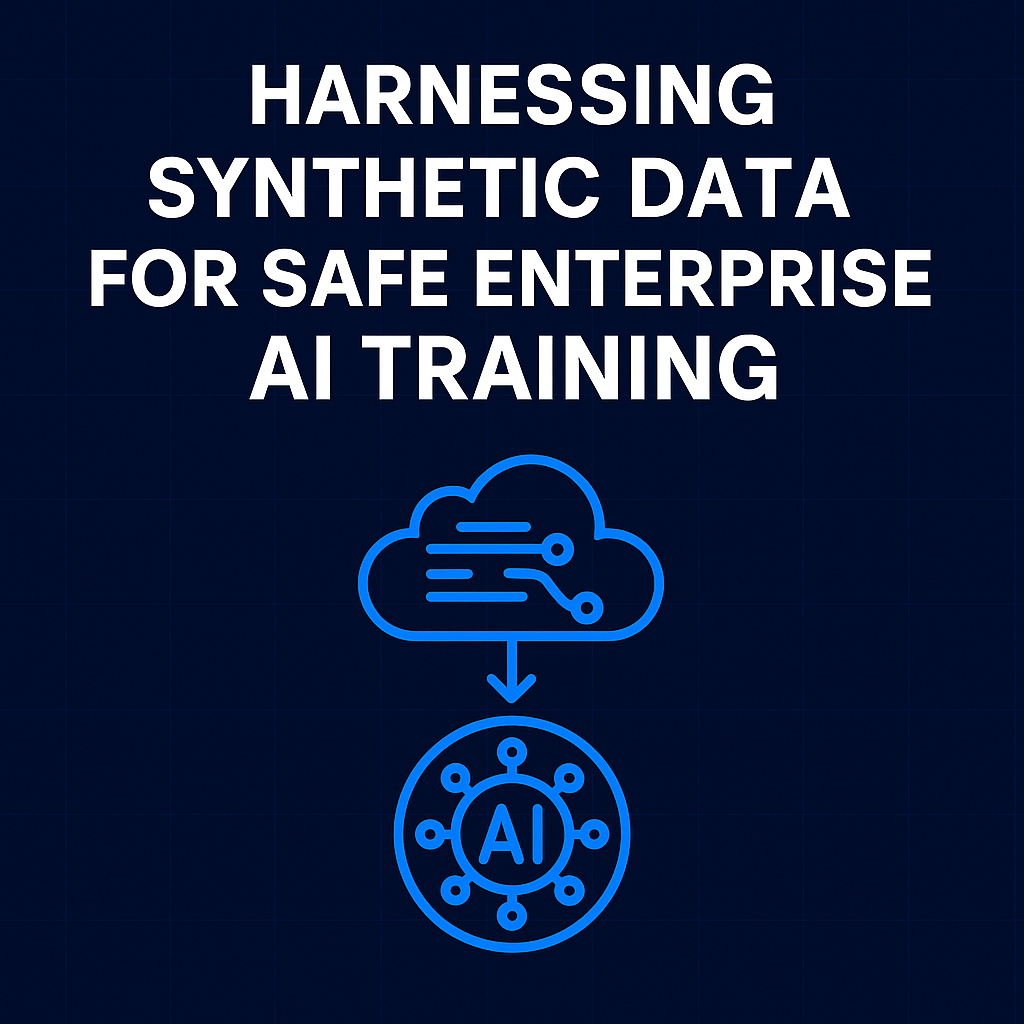
Artificial Intelligence (AI) systems thrive on data, clean, abundant, and diverse datasets are essential for effective model training and performance. In enterprise environments, however, accessing real-world data often comes with limitations. Privacy regulations, data scarcity, and the risk of exposing sensitive customer or operational information present ongoing challenges. To overcome these barriers while maintaining AI development velocity, organisations are increasingly turning to synthetic data.
Synthetic data, artificially generated information that mimics real-world datasets, emerges as a transformative asset in enterprise AI. It enables safe, scalable, and bias-controllable training of AI models without compromising security or compliance.
The Role of Synthetic Data in Enterprise AI
Enterprises deal with vast amounts of structured and unstructured data, much of which resides in regulated or siloed environments. Customer data, financial transactions, healthcare records, and proprietary industrial insights are often inaccessible for experimentation. Synthetic data steps in to simulate these complex scenarios without the risks associated with using actual data.
Generated using statistical models, generative adversarial networks (GANs), or other AI-driven algorithms, synthetic data replicates the patterns, structure, and variability of real-world datasets. When trained on such data, AI systems learn to generalise effectively, often with better privacy protection and improved control over edge cases.
Key Benefits of Synthetic Data in the Enterprise
1. Data Privacy by Design
In industries such as finance, healthcare, and telecommunications, data privacy is a non-negotiable priority. Synthetic data eliminates the need to expose personally identifiable information (PII) or customer details during the training of AI models. Since it contains no real user records, synthetic datasets inherently support GDPR, HIPAA, and other compliance standards.
This shift empowers teams to build AI solutions faster, with governance teams confident that sensitive data remains protected.
2. Accelerated AI Model Development
Generating synthetic data removes bottlenecks caused by limited data access or time-consuming anonymisation. AI and data science teams can instantly produce datasets tailored to specific training needs, such as edge cases, rare anomalies, or underrepresented demographics.
This accelerates the experimentation cycle, supporting faster model prototyping, iteration, and refinement, which in turn shortens the time to value in enterprise AI initiatives.
3. Balanced and Bias-Controlled Training
Real-world data often contains biases, such as imbalances in gender, geography, usage patterns, or historical trends, that AI models may learn and reinforce. Synthetic data offers an opportunity to deliberately rebalance datasets.
Teams can generate balanced samples that represent diverse user profiles or correct for skewed class distributions. This results in AI systems that are more inclusive, fair, and robust in deployment.
4. Safe Testing and Simulation Environments
Enterprise AI applications benefit from rigorous testing before going live. Synthetic data allows teams to simulate operational environments—stress-testing models under various conditions, edge cases, or failure scenarios.
For example, banks can simulate fraudulent transactions, logistics firms can model rare delivery disruptions, and hospitals can create synthetic patient journeys. These scenarios help refine model responses and ensure that the model performs resiliently in production.
Real-World Enterprise Applications
- Banking and Finance: Synthetic transaction data supports the training of fraud detection models without requiring access to real accounts or transaction logs.
- Healthcare: AI models trained on synthetic patient records help with diagnostics, without compromising the confidentiality of real patients.
- Retail: Purchase behaviours and product interaction simulations train recommendation engines and inventory algorithms.
- Manufacturing: Simulated sensor data from industrial equipment helps predictive maintenance systems learn failure patterns safely.
Challenges to Address
While synthetic data offers transformative potential, its effectiveness depends on the quality of generation and validation. Poorly generated data may lack the variability or realism required for accurate training. To address this, enterprises combine synthetic data with real data in hybrid approaches, ensuring both performance and safety.
Another challenge is monitoring model performance after deployment. Even with excellent training, synthetic data cannot capture the full complexity of real-world variability. Continuous monitoring and retraining with updated data remain critical.
Best Practices for Using Synthetic Data in AI Training
- Select the Right Generation Method: Choose between rule-based generation, GANs, or variational autoencoders depending on the complexity of the data needed.
- Validate Against Real Data: Compare model performance on synthetic versus real datasets to measure training efficacy.
- Prioritise Diversity: Ensure synthetic data includes sufficient edge cases, minority categories, and rare patterns.
- Monitor in Production: Track accuracy and drift of models trained on synthetic data, and retrain as needed with fresh data.
- Combine with MLOps Pipelines: Integrate synthetic data workflows into broader MLOps practices to manage lifecycle and governance.
As enterprise AI matures, the use of synthetic data will become a standard practice across industries. It offers a reliable pathway to train, test, and deploy models safely, especially in environments where real-world data is sensitive, scarce, or slow to access. The combination of speed, compliance, and model fairness makes synthetic data a critical enabler of scalable, responsible AI.
Organisations that invest in synthetic data capabilities today will lead tomorrow’s innovation—unblocking creativity, safeguarding trust, and accelerating AI value creation.
Leave a Reply