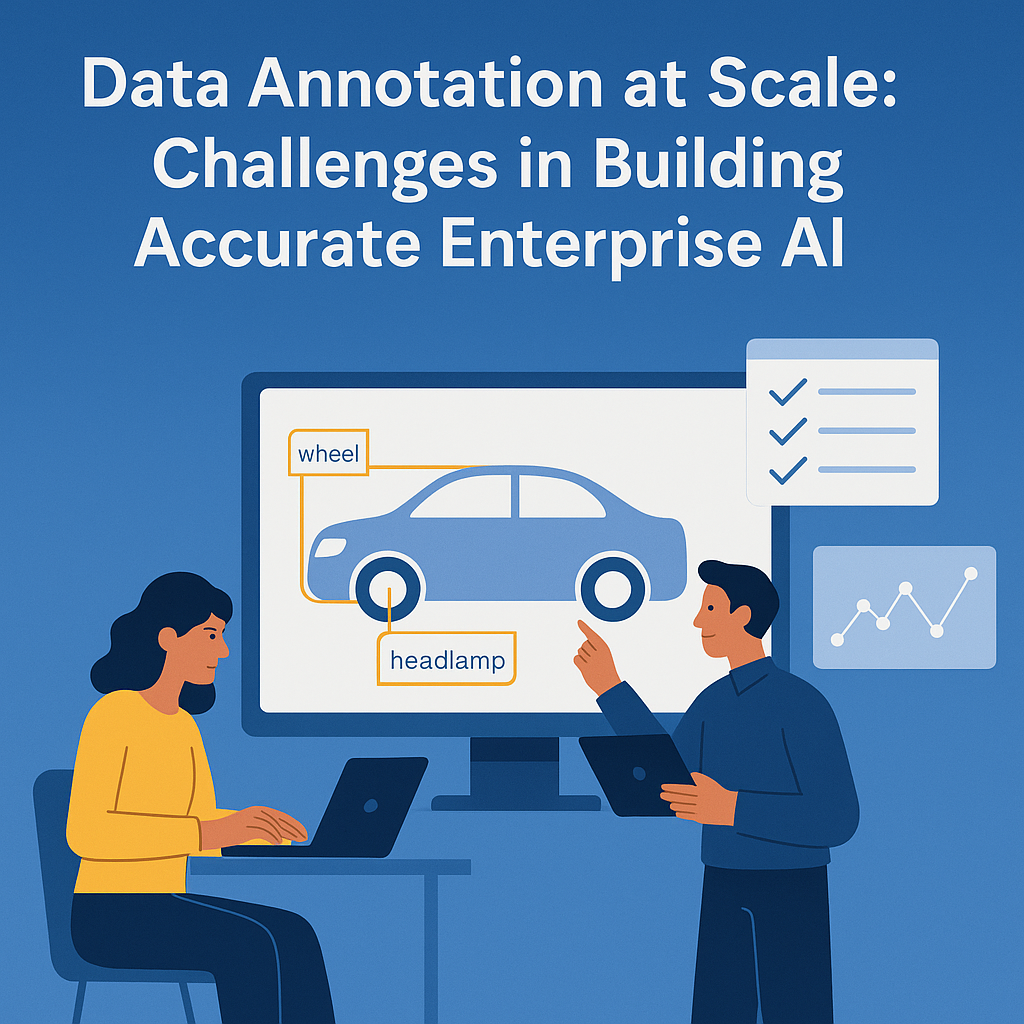
As artificial intelligence (AI) continues to revolutionise industries, one of the most crucial yet often overlooked components in building robust AI systems is data annotation. Whether it’s for training machine learning models, enhancing natural language processing (NLP) algorithms, or improving computer vision systems, the accuracy and quality of annotated data directly impact the performance of AI systems.
For enterprises, the task of data annotation at scale presents a unique set of challenges, particularly in maintaining high-quality datasets while managing the vast volume of data generated daily.
What is Data Annotation?
Data annotation is the process of tagging or labelling data to make it useful for AI and machine learning algorithms. This could involve anything from categorising images, tagging speech in audio files, labelling text for sentiment analysis, to annotating video footage for object recognition.
For AI models to learn, they need to be trained on labelled datasets that help them understand and predict outcomes. This annotation is crucial because it directly feeds into the model’s ability to make accurate predictions or classifications.
Why Data Annotation Matters for AI
Data annotation serves as the bridge between raw, unstructured data and the machine learning models that drive AI. Without accurate annotations, AI systems cannot accurately identify patterns or make meaningful predictions, rendering them ineffective. For example, in autonomous vehicles, incorrect or incomplete data annotation can lead to errors in object detection, potentially resulting in unsafe driving decisions.
In enterprise AI projects, data annotation serves several key purposes:
- Model Accuracy: The quality of data directly correlates with the AI model’s performance. Better-annotated data means a more accurate model.
- Customisation: Enterprises often need tailored AI models that cater to their specific needs. High-quality annotated data allows organisations to fine-tune algorithms for particular tasks or industry-specific requirements.
- Compliance and Ethical AI: Well-annotated data can help AI systems avoid biases, meet ethical standards, and comply with regulatory frameworks (e.g., GDPR, HIPAA), as accurate data helps prevent discriminatory outcomes.
Challenges in Scaling Data Annotation for Enterprises
1. Volume of Data
One of the most significant challenges in data annotation is the sheer volume of data. With enterprises collecting vast amounts of data daily, scaling annotation processes to handle this flow while maintaining high quality becomes a herculean task. For example, a video streaming company might need to annotate millions of hours of video data to enhance its content recommendation engine.
- Solution: Leveraging AI-assisted annotation tools can speed up the process by automating repetitive tasks and reducing the workload on human annotators. AI systems can pre-annotate data, allowing human experts to validate and refine these annotations, significantly improving efficiency.
2. Maintaining Consistency and Quality
For AI models to learn effectively, annotations must be consistent across large datasets. Variability in how annotators label data can introduce errors, causing the model to develop inaccurate patterns. This becomes even more difficult when multiple annotators with varying expertise and understanding are involved.
- Solution: Standardising the annotation process with clear guidelines, training materials, and quality assurance protocols can mitigate this issue. Platforms that allow multiple annotators to work together and resolve discrepancies through a consensus mechanism can also improve consistency.
3. Human Resource Constraints
Data annotation at scale requires a large and skilled workforce, especially for complex tasks such as sentiment analysis, object recognition, or medical image annotation. While it’s possible to hire large teams of annotators, the quality of the annotations can decline over time due to fatigue or a lack of expertise.
- Solution: Crowdsourcing platforms and specialised annotation services can help address this challenge by tapping into a broader talent pool, but proper oversight and training are essential to ensure quality. Additionally, AI tools that streamline the process can reduce the need for human workers to perform repetitive tasks.
4. Cost and Time Constraints
The process of annotating data, particularly at scale, can be both time-consuming and expensive. For enterprises, this can mean significant resource allocation that could otherwise be used to develop the AI application itself. Ensuring that annotations are completed in a timely and cost-effective manner is critical for enterprises looking to deploy AI quickly and efficiently.
- Solution: Using semi-automated and AI-assisted annotation tools can help speed up the process while maintaining quality, reducing both costs and time. Additionally, outsourcing or leveraging remote teams can reduce overheads.
5. Data Privacy and Security
In many industries, such as healthcare and finance, annotated data often contains sensitive information, and mishandling or exposure of this data can result in serious privacy breaches. Ensuring that the annotation process adheres to privacy laws and organisational security policies is crucial for enterprises working with personal or proprietary information.
- Solution: Encrypted annotation platforms, secure cloud storage, and a strict data access control policy can ensure that sensitive information is protected throughout the annotation process. Additionally, enterprises should provide their teams with appropriate training on data privacy best practices.
6. Scalability of Annotation Infrastructure
Building a scalable infrastructure to handle the massive amounts of data that need to be annotated across different formats (text, image, video, etc.) can be complex. Traditional methods, such as using spreadsheets or manual data entry, don’t cut it when dealing with big data.
- Solution: Cloud-based annotation tools, which offer scalability and flexibility, are increasingly popular in enterprise settings. These platforms enable rapid scaling to meet the needs of large datasets, and their ability to integrate with AI tools can create a seamless pipeline from annotation to training.
Best Practices for Scaling Data Annotation
- Invest in the Right Tools: Leveraging AI and automation for data pre-processing and annotation can reduce manual effort and improve consistency.
- Create Detailed Guidelines: Clear instructions and standardised protocols for annotators can significantly reduce errors and ensure consistency.
- Use a Hybrid Approach: Combine human expertise with machine learning models for semi-automated annotation. This approach enhances the accuracy of human annotators by leveraging AI’s ability to handle repetitive tasks.
- Ensure Continuous Feedback: Regularly validate and update the dataset to refine AI models based on real-world feedback. This approach helps improve the overall quality of the annotations.
- Prioritise Data Privacy: Implement strict security measures to protect sensitive data and comply with relevant data protection regulations.
Conclusion
Data annotation at scale is one of the most critical tasks in building accurate and effective AI models, especially for large enterprises. While it poses challenges in terms of volume, consistency, cost, and privacy, these obstacles can be overcome with the right combination of human expertise, automated tools, and strategic planning.
For enterprises looking to leverage AI at scale, investing in the right infrastructure for data annotation is essential. By taking a systematic approach and adopting best practices, businesses can ensure that their AI models are built on high-quality data, leading to more accurate predictions, improved decision-making, and ultimately, a competitive edge in their respective industries.
Leave a Reply